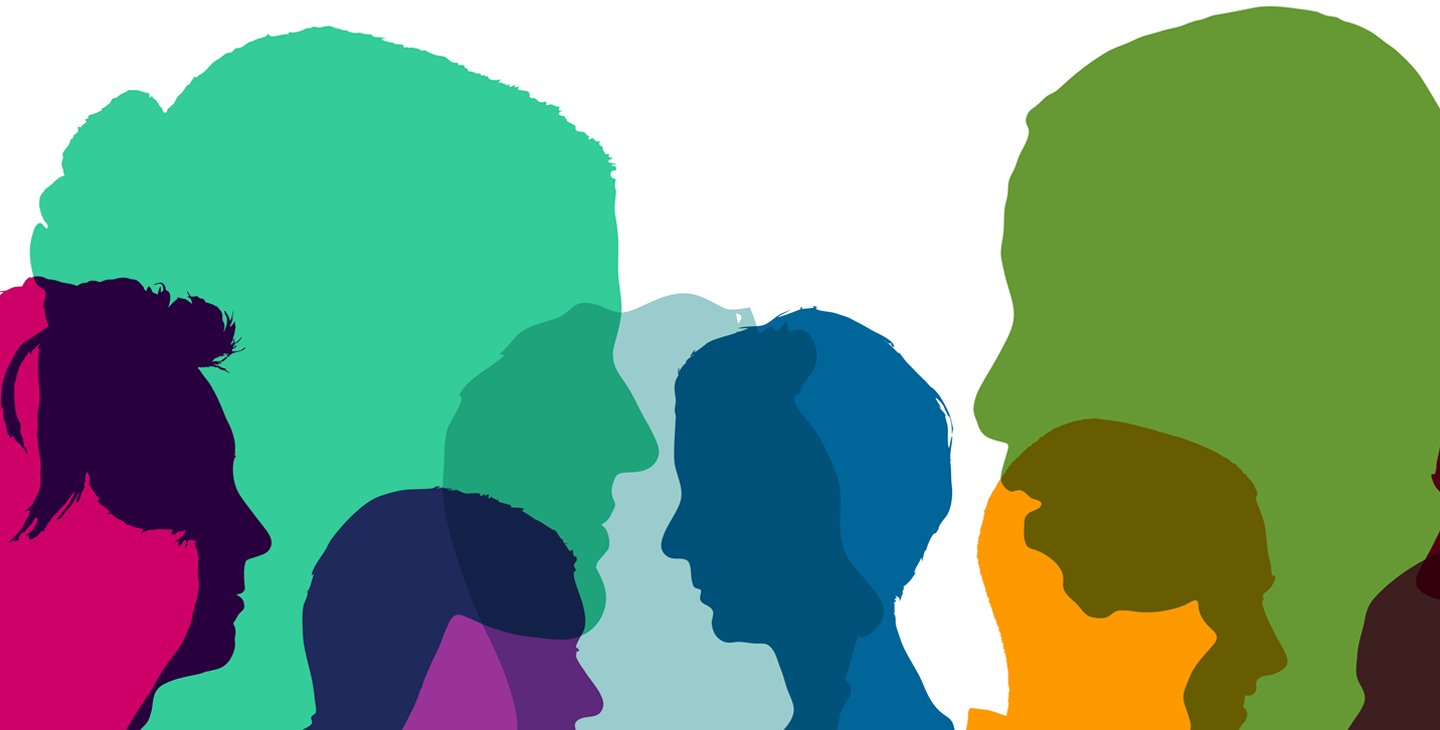
How Do You Study Facial Bias Without Bias?
When we encounter an unfamiliar face, we tend to make snap judgments. Does the person look smart, attractive, or young? Are they trustworthy or corrupt? Neuroscientists and psychologists study how our brains form these facial biases, and how the judgments ultimately influence the way people behave.
"We tend to be quite confident in the judgments we make based on people's faces, but we're often wrong," says Ralph Adolphs (PhD '93), Bren Professor of Psychology, Neuroscience, and Biology and an affiliated faculty member of the Tianqiao and Chrissy Chen Institute for Neuroscience.
Previous studies have linked these stereotypes and judgments to the decisions people make in various aspects of society, including elections, hiring practices, and court sentencing by juries. For instance, a Caltech study from Adolphs and Mike Alvarez, a political science professor, showed that people judged politicians to be more corrupt if they had wider faces, and that, in this case, these judgments coincided with whether the politicians had been convicted of corruption in real life.
"Very important social decisions are influenced by the snap judgments that we make about people from their faces," says Adolphs. "By pointing out these biases, we hope that we can reduce their impact."
In one recent study in the journal Nature Communications, Adolphs and his team, led by former Caltech graduate student Chujun Lin, now a postdoctoral fellow at Dartmouth College, looked at how facial biases can be broken down into primary judgments. In the same way that the multifaceted colors of a painting can be derived from the primary colors of red, yellow, and blue, our brains blend primary judgments together to create an array of perceptions about everything from how kind a person is to their levels of aggression.
The results showed that the study participants, which included people from seven different regions around the world, automatically made four primary judgments when encountering a new face (regardless of whether the judgments were accurate or not): they assessed whether a person is warm or cold, competent or incompetent, feminine or masculine, and young or old. All other judgments people may make can be derived from a mix of these four primary judgments.
"These four primary judgments underlie the biases we hold when forming a wide range of impressions of others based on faces, which could be targeted efficiently for anti-bias interventions," Lin explains.
Challenges to Studying Bias
Adolphs notes there are limits to this particular study and many others like it. Here, the researchers used existing databases, which are largely comprised of white faces with neutral expressions.
"Most of the databases for these types of studies were constructed years ago, and even decades ago," says Adolphs. "There typically are photos of people readily available to the investigators, but the photos certainly do not represent the world's population."
For their initial analysis, Adolphs and his team chose to limit the stimuli to white faces with neutral expressions because this allowed them to exclude other factors such as context and race. The team is working on a follow-up project that brings in more diverse faces, including faces of different races that exhibit a broader range of expressions.
"Representing the diversity of a general world population is a big challenge in our field," says Adolphs.
A seminal University of British Columbia study on the issue, says Adolphs, introduced a term known as WEIRD, for Western, Educated, Industrialized, Rich, and Democratic societies. WEIRD refers to populations of people typically studied in psychology and social science. As the article points out, "this particularly thin, and rather unusual, slice of humanity," is one of the "least representative populations one could find for generalizing about humans."
"For a lot our studies, we don't recruit students for this reason," says Adolphs. "They are convenient, but they are of course not a representative demographic subsection of the world's population. Often, we try to recruit people from the community who are more diverse."
The future: bias in AI
In another recent study from Adolphs' group, led by Caltech postdoc Umit Keles and published in the journal Affective Science, the researchers looked at the question of whether artificial intelligence (AI) methods can be trained to predict how individuals will react to people's faces. They found machine-based methods could make surprisingly accurate predictions, but sometimes came up with wrong answers.
"A round face might look baby faced and kind, but also corrupt, depending on the details. Because the features in faces are so closely related to one another, you can get many kinds of misjudgments from these algorithms," says Keles. "There is a worrisome potential for misuse of these AI methods."
This past summer, a Summer Undergraduate Research Fellowship (SURF) student in Adolphs' lab, Leena Mathur, worked on a project that examined how AI models might be trained to perceive human emotions across cultures. She used videos of people talking to each other from a database created by researchers at Imperial College London. The database includes people from six cultures: British, Chinese, German, Greek, Hungarian, and Serbian. The preliminary findings suggest AI models can be trained on videos of people communicating in one cultural context and subsequently adapted to detect emotions from videos of people communicating in other cultural contexts.
"There is a field-wide effort to collect more diverse data for AI research," she says. "The goal is to ultimately develop AI systems that are inclusive and can support people across race, age, gender, culture, and every other dimension of human diversity."
Mathur, a student at USC, hopes her research will eventually contribute to AI systems that support human health and societal well-being across cultures.
"There is potential for misuse of these technologies, so it is important to research how robots and AI systems can be effectively adapted across cultural contexts for assistive applications," she says.
Adolphs says his team's lab meetings always include discussions on diversity and racism (the lab has a Diversity, Equity, and Inclusion representative, postdoc Nina Rouhani).
"It's a topic we continue to be very concerned about. We talk about all of these issues and ask ourselves, 'What else can we do?' We are continuing to emphasize issues of race and representativeness in our science."
More about the team's guiding principles can be found on the lab website.