Developing Realistic Models of Financial Markets: A Conversation with Lawrence Jin
Lawrence Jin (MS '06) is a new assistant professor of finance at Caltech. Born in Beijing, China, Jin studied physics and mathematics at Tsinghua University, earning bachelor's degrees in both fields in three years. In 2005, he came to the United States and earned a master's degree in electrical engineering at Caltech. Then he tried something completely different, spending a few years working as a research and trading analyst on Wall Street. Ultimately, he opted to pursue academic finance and earned his doctorate in financial economics at Yale University.
Jin's arrival at Caltech marks an important step in building a finance faculty to support Caltech's Business, Economics, and Management (BEM) option and to expand the research activities of the Ronald and Maxine Linde Institute of Economic and Management Sciences. In the spring term, Jin taught courses in Behavioral Finance (BEM 114) and Asset Pricing Theory (SS 215).
We recently sat down with Jin to discuss the types of problems he is interested in, how psychology and neuroscience can help inform financial models, and what brought him back to Caltech.
What is the focus of your research?
My main research area is behavioral finance, a very active field within the broader field of economics and finance. We try to develop psychologically plausible and realistic models to better understand financial markets.
When you say "behavioral" in this context, what do you mean?
Actual human behavior. For instance, people are not necessarily making fully rational decisions using all the available information they can possibly obtain; they cannot pay attention to every single thing they encounter. And they have some psychological biases when forming opinions of the financial market.
The key questions are: In what way are people systematically irrational? And how can we model irrationality and its interaction with other economic forces to better understand financial markets? When addressing these questions, we try to discipline ourselves by understanding people's behavior through the lens of psychology, behavioral sciences, biology, and neuroscience.
Can you give some examples of the types of problems you study?
One example is to understand stock market fluctuations and other asset pricing phenomena through the lens of psychological biases.
One type of psychological bias is something called sample-size neglect, the notion that many investors mistakenly think small samples can be just as representative as large samples. In other words, investors tend to draw a conclusion too quickly. For instance, if you see a sequence of good stock market returns, it may just be random. But if you think that you are actually seeing a trend, you might positively revise your expectations of future returns. And it turns out that you revised your expectations too quickly. This is an example that links sample-size neglect to a finance application.
It is important to note that the behavioral approach to study financial markets is not an isolated approach. Sometimes it interacts with other things like financial frictions.
What are financial frictions in this context?
In financial markets, there are many frictions. For instance, ordinary households typically do not invest directly in opaque markets such as the market of mortgage-backed securities. Instead, they invest through mutual fund managers. With this specific structure, some conflicts of interest may arise. For example, mutual fund managers might care more about making money for themselves than helping their clients. Such frictions ultimately could interact with behavioral biases, and they can amplify each other, especially during bad times like financial crises.
Another example is transaction costs that you need to pay a broker when you buy and sell stocks and bonds. One finding in finance is that when individual investors decide to actively manage their own stock portfolios, on average, they underperform. In other words, if you instead give your money to an index fund, you are likely to do better.
Financial economists try to understand why individual investors trade so much on their own even though they underperform index funds.
One behavioral explanation is that investors may be overconfident in their ability to invest. There is a very nice paper by Mark Grinblatt [UCLA Anderson School of Management] and Matti Keloharju [Aalto University School of Business in Finland] that uses data from Finland to show that people with a higher level of overconfidence trade more. In Finland, all of the 18-year-old male citizens are required to go into the military. When they do so, they take aptitude tests and behavioral tests. Overconfidence in this paper is measured as their self-reported confidence based on the behavioral tests minus how confident they should be based on their performance on the aptitude tests. The interesting thing is that this measurement of overconfidence predicts how frequent people trade stocks several years later when they open their brokerage accounts. And those who are more overconfident trade more and have poorer trading performance.
Understanding behavioral biases such as overconfidence is helpful not only for understanding financial markets, but also for helping people to make better decisions—things like saving more money for retirement and keeping their jobs.
Can you share some results from some of your recent work?
I am very interested in understanding the origin of financial bubbles and crashes. We study why bubbles—for instance, housing bubbles—arise in the first place and also, along with the formation of bubbles, why people begin to trade more. How long is a bubble going to last? When and why do bubbles eventually crash? And what are the consequences?
Our model is trying to answer these questions through something called extrapolative expectations. Consistent with the sample-size neglect we discussed earlier, extrapolative expectation is the notion that after seeing a sequence of good stock returns, many real-world investors tend to believe that the stock market is going to keep rising in value
In this model, a fundamental shock is needed for a bubble to start—something like good news about the market. Those signals create a positive price impact on the market, so market prices go up. Then our extrapolators, people who have these extrapolative expectations, start to get more and more excited. They take the initial increase in market prices too seriously, and their self-enforcing beliefs end up helping prices to keep going up. However, as the initial good news that got people excited in the first place recede into the distant past, extrapolators' irrational exuberance diminishes, and the whole bubble unravels—you get a crash.
How does the idea of frenzied trading come into play?
There is lots of empirical evidence that suggests when bubbles occur, you see a lot of trading in financial markets: investors buy and sell lots of stocks. Economists have had a hard time explaining this.
We have this idea, supported by some neuroscience studies, that when investors are looking at a stock market as a bubble is being created, their trading decisions are influenced by two conflicting signals. On the one hand, investors see a positive trend, and their extrapolative expectations tell them that the price is going to keep going up. This is what we call a growth signal. On the other hand, investors are also aware of the fact that the stock market may be overvalued, and therefore it may crash in the near future. We call this a value signal. Given that the value signal and the growth signal typically tell investors to trade in the opposite direction, investors may slightly change the weights they put on these conflicting signals over time¾we call these changes in weight "wavering."
As the bubble develops, these two signals endogenously become very large, or extreme.
And as a result, a small degree of wavering could generate a lot of trading volume.
Intuitively, you can think of the value signal and growth signal as two voices in your head telling you different things. During normal periods, the voices are speaking in pretty low tones. In this case, you may be wavering, but that does not change your actions much. But during bubble periods, it is like you have two crazy voices screaming at you, telling you radically different things. Then even the same small degree of wavering is going to cause lots of trading.
The idea of wavering we came up with turns out to be very helpful in generating lots of trading volume during bubbles.
Do you plan to collaborate with anyone in particular at Caltech?
On the one hand, my research is very structural and mathematical. On the other hand, it requires very good intuition about financial markets. To get that intuition right, sometimes you need to work with psychologists, neuroscientists, biologists, and behavioral economists. Caltech has a lot of strength in these other areas that can definitely help me to build my research.
Were there any other factors that led you back to Caltech?
I really like the idea of having an impact on talented students, and Caltech clearly has very high-quality undergraduate and graduate students. I think it is going to be fun to teach the students and do research with them.
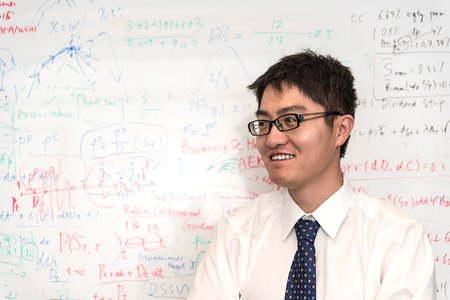
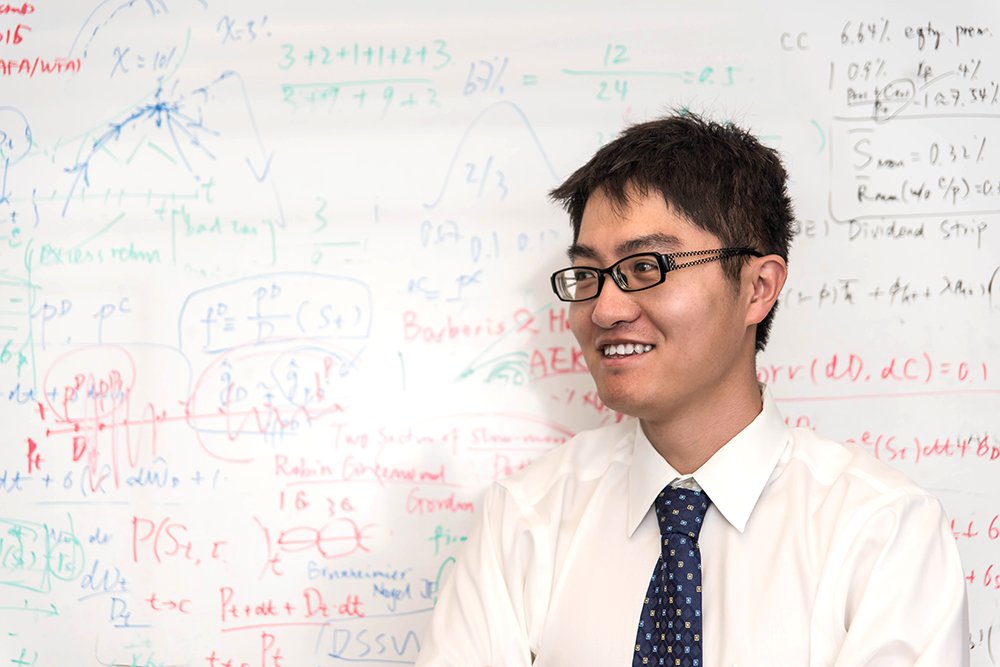